
Surface-Engineered Graphene surface-enhanced Raman scattering Platform with Machine-learning Enabled Classification of Mixed Analytes
This is an Open Access article distributed under the terms of the Creative Commons Attribution Non-Commercial License(https://creativecommons.org/licenses/by-nc/3.0/) which permits unrestricted non-commercial use, distribution, and reproduction in any medium, provided the original work is properly cited.
Abstract
Surface-enhanced Raman scattering (SERS) enables the detection of various types of π-conjugated biological and chemical molecules owing to its exceptional sensitivity in obtaining unique spectra, offering nondestructive classification capabilities for target analytes. Herein, we demonstrate an innovative strategy that provides significant machine learning (ML)-enabled predictive SERS platforms through surface-engineered graphene via complementary hybridization with Au nanoparticles (NPs). The hybridized Au NPs/graphene SERS platforms showed exceptional sensitivity (10-7 M) due to the collaborative strong correlation between the localized electromagnetic effect and the enhanced chemical bonding reactivity. The chemical and physical properties of the demonstrated SERS platform were systematically investigated using microscopy and spectroscopic analysis. Furthermore, an innovative strategy employing ML is proposed to predict various analytes based on a featured Raman spectral database. Using a customized data-preprocessing algorithm, the feature data for ML were extracted from the Raman peak characteristic information, such as intensity, position, and width, from the SERS spectrum data. Additionally, sophisticated evaluations of various types of ML classification models were conducted using k-fold cross-validation (k = 5), showing 99% prediction accuracy.
Keywords:
Graphene, Surface engineering, Surface-enhanced Raman scattering (SERS), Machine learning1. INTRODUCTION
Surface-enhanced Raman scattering (SERS) exploits diverse surface interactions between the material under analysis and the metal surface, yielding an amplified Raman signal beyond typical Raman spectroscopy. SERS shows surface signal enhancements in the range of 1014–1015 in magnitude, enabling detection down to the level of single molecules [1,2]. This remarkable capability stems from its distinctive vibrational properties, allowing rapid and precise detection of structural information in both chemical and biological molecules. SERS finds applications in trace detection of hazardous substances, quantitative determination of molecular concentrations, and flow cytometry, outperforming conventional Raman spectroscopy. Moreover, it is an effective tool for identifying and characterizing biological and chemical molecular components, revealing their compositions, structures, aggregation states, and ligand-binding effects [3-6]. The chemical mechanism underlying SERS operates at the molecular level, where the proximity between the substrate and molecule induces charge transfer. This phenomenon significantly alters the charge distribution of the molecule, enhancing its polarizability, and consequently, the cross-section of Raman scattering. In addition, the amplification of the local electromagnetic field, triggered by surface plasmons excited by incident light, represents a long-range effect that necessitates substrate roughness, emphasizing the significance of hotspot generation. In this regard, the pivotal factor for achieving substantial SERS enhancement lies in obtaining a nanometrically hybridized or surface-engineered conformal substrate. Traditionally, SERS substrates rely on the rough surfaces of noble metals such as Ag, Au, and Cu. These substrates interact with nearby molecules, including surface plasmon resonance that generates hot spots when irradiated with light at specific frequencies. Within these hotspots, a local electric field is generated, intensifying the Raman signal [7-11]. However, as detection sensitivity in analytical technology advances, various interferences may arise between the detection material and analyte equipment.
Conventional SERS encounters challenges as the detection material directly contacts the precious metal surface, particularly metals such as Au and Ag, which catalyze various oxidation/reduction reactions, resulting in diverse chemical bonds on the SERS substrate and subsequent reactions. This significantly compromises the integrity of the SERS spectrum, final detection sensitivity, and material characterization [12]. To overcome this limitation, surface-engineered graphene has been introduced. Its smooth surface lacks hot spots, primarily generating SERS phenomena through chemical interactions with target analytes. Moreover, by introducing noble metal nanoparticles (NPs) onto the graphene surface, a significant enhancement in the electromagnetic interactions can be anticipated, along with additional chemical interactions and exceptional chemical stability originating from the graphene. Furthermore, the π–π bonds within graphene serve as a local magnet, providing a binding force for target analytic molecules, thereby enhancing detection sensitivity. [13-23]. Because of the complexity of analyzing SERS signals and the presence of numerous signal overlaps, data analysis approaches combined with machine learning (ML) have focused on identifying and distinguishing the Raman spectral features of various molecular components among their similar chemical forms from the complexity of the spectrum [24-27].
Here, we demonstrate surface-engineered graphene-based SERS platforms achieved through complementary hybridization with Au NPs. The structural and chemical features of the synthesized SERS platforms were systematically investigated through microscopic and spectroscopic analyses. Our SERS platform showed exceptional detection sensitivity for various analytical molecules (Rhodamine 6G (R6G), Methylene Blue (MeB), and Sudan I), boasting extraordinary detection limits of 10-7 M. In addition, in collaboration with our data analysis approach, we developed an artificial intelligence classification model demonstrating 99% prediction accuracy. This was achieved by optimizing the hyperparameters for our proposed neural network classification model. These ML models hold promise for further classification of mixed analytical molecules.
2. EXPERIMENTAL
2.1 Preparation of SERS substrates
The synthesized graphene was transferred onto a SiO2/Si substrate following a multistep process. Initially, graphene was grown on Cu foil using the conventional thermal chemical vapor deposition method. For transfer onto a SiO2/Si substrate, a poly (methyl methacrylate) (PMMA)-assisted wet transfer technique was employed. The graphene film was spin-coated with PMMA (500 rpm for 5 s and 2000 rpm for 40 s), facilitating its adherence to the substrate. Subsequently, the Cu foil was etched using an aqueous Cu etchant, resulting in a PMMA/graphene film. This film was thoroughly washed with deionized (DI) water and then transferred onto the SiO2/Si substrate. After complete drying of the substrate, the PMMA layer was removed using acetone, leaving the graphene film atop the SiO2 layer. Following the transfer process, Au NPs were prepared as follows: 99.99% pure Au grains served as the source material for thermal evaporation, which was performed in an electrically heated tungsten boat. The prepared graphene/SiO2 substrate was then placed into a thermal evaporator for the deposition of 3 nm-thick Au NPs at a rate of 0.1 Å/sec.
2.2 Characterization
The chemical identification of pristine graphene films and Au NPs–graphene hybrid films was conducted using X-ray photoelectron spectroscopy (XPS, K-Alpha, Thermo Fisher Scientific) with conventional monochromatic Al Kα radiation (hν = 1486.6 eV). Structural characterization was performed using scanning electron microscopy (SEM, S-4700, Hitachi), and Raman spectroscopy (InVia Raman microscope, Renishaw) coupled with an optical microscope (BX53MTRFS, Olympus). For SERS analysis, aqueous solutions of R6G, MeB, and Sudan I (1×10-7 M concentration) diluted in DI water were dropped directly and dispersed onto the surface of the prepared substrates. After the droplets were dried under ambient air conditions, Raman measurements were conducted within the spectrum range of 550–2050 cm-1. Raman spectra were recorded at an excitation wavelength of 532 nm within an intensity of 30 mW/cm2.
2.3 Data preparation for ML
An ML database was established using the SERS spectrum data obtained from diverse substrates. For streamlined data analysis, characteristic features such as peak positions, widths, and intensities were extracted from each Raman spectrum. ML tasks were conducted using MATLAB, incorporating robustness measures and mitigating overfitting by employing k-fold cross-validation (k = 5) during the training phase. In this MATLAB-based framework, model performance was assessed using performance metrics such as Mean Absolute Error (MAE), Root Mean Square Error (RMSE), etc., to evaluate accuracy and predictive capabilities.
3. RESULTS AND DISCUSSIONS
Fig. 1 (a) illustrates a schematic of the SERS substrate, described as Au NPs/graphene/SiO2/Si. The fabrication process involved transferring graphene onto a cleaned 300 nm-thick SiO2/Si (001) substrate using a wet transfer method assisted by PMMA. Subsequently, 3 nm-thick Au NPs were deposited onto the graphene/SiO2/Si substrate using a conventional thermal evaporation technique. This process resulted in the creation of a hybrid SERS substrate exhibiting high uniformity over a large area. Each Au NP contributes to the enhancement of Raman scattering through its plasmonic electric field, whereas graphene plays a crucial role in enhancing Raman scattering via charge-transfer mechanisms. In Fig. 1, top-view and cross-sectional SEM images are presented. Fig. 1 (b, c) show a clean and uniform surface of the Au NPs/graphene SERS platforms, showcasing Au NPs with a few-nanometer size. Based on these observations, we concluded that the defective sites of graphene were decorated with Au NPs, uniformly distributed across the graphene surface, with an average size of 3 nm. This indicates that wrinkles in graphene could potentially serve as activation sites for the nucleation and formation of Au NPs. In addition, the spacing observed in this particular substrate was only a few nanometers, indicating the potential for establishing a much stronger electromagnetic field on the surface-engineered SERS platform. XPS analysis was conducted using conventional monochromatic Al Kα radiation (hν = 1486.6 eV) to elucidate the chemical state of the substrate surface. The XPS survey, C 1s, and Au 4f core-level spectra of pristine graphene and Au NPs/graphene hybrid films are shown in Fig. 1 (d), and their atomic percentages are summarized in Fig. 1 (e). Upon the decoration of Au NPs on the surface of graphene, a noticeable decrease in the intensity of the C 1s peaks was unequivocally observed, attributed to the reduction of the exposed carbon surface. Analysis of the C 1s core-level spectra for the graphene and Au NPs/graphene SERS platforms revealed a predominant sp2 C–C component (binding energy, EB = 284.5 eV) and negligible oxygen-containing components such as C–O, C=O, and O=C–O. These findings indicate that the chemically identical state associated with the graphene film remained consistent irrespective of the presence of Au NPs. The presence of pure Au NPs was confirmed through the Au 4f core-level analysis. In the case of the Au NPs/graphene hybrid films, peaks within the binding energy range of 83–88 eV can be attributed to the Au 4f core-level spectra originating from the Au NPs. This information indicates the formation of a homogeneous composite of graphene and Au NPs. Raman spectroscopy was conducted to evaluate the SERS enhancement of the Au NPs/graphene hybrid films, as shown in Fig. 1 (f). The Raman spectrum of pristine graphene exhibited three distinctive peaks: the D-band (~1350 cm-1), G-band (~1580 cm-1), and 2D-band (~2650 cm-1). Specifically, the G band is attributed to ordinary first-order resonance Raman scattering. These scattering results involve the degenerate zone-center optical phonon mode with E2g symmetry (iLO and iTO), which is consistent with the electrons at the I- point. Notably, the G band can be used to estimate the doping concentration due to the Kohn anomaly suppression with changing Fermi energy. In contrast, the 2D-band arises from second-order double-resonance Raman scattering involving two iTO phonons near the K point. Since the 2D-band reflects the electronic band structure of graphene, it helps in investigating the electronic structure of graphene and perturbations in graphene, such as interlayer interactions, number of graphene layers, and strain. The D-band appears from a different intervalley double-resonance Raman process related to an electron at the K point. This predominantly occurs when defects are present in the graphene structure. All of these Raman contributions were also evident in the Au NPs/graphene hybrid films; however, some relevant differences were identified. Through the integration of graphene and Au NPs, the Raman intensities of all peaks showed significant enhancement compared to those of pristine graphene, attributed to a certain degree of disorder induced by Au NP insertion within the graphene layer. The strong excitation of surface plasmons induced by the metallic NPs determines the overall change in the dipole moment during vibration, even in the absence of a polarizability change. The distinct enhancement of the G-band primarily stems from the electromagnetic effect arising from the charge transfer between Au NPs and the π electrons of graphene. Furthermore, alteration of the electronic band structure of graphene results in an increase in the full width at half the maximum of the peaks. The enhancement of SERS in the Au NPs/graphene hybrid film was more pronounced in the G-band than in the 2D-band, indicating the susceptibility of the G-band to SERS. Another contributing factor to the strong Raman enhancement may be the better superposition of the plasmon resonance of the SERS substrates with the excitation line of 532 nm used in the experiments. When the plasmonic wavelength of the SERS substrate resonates with the excitation, a highly intensified Raman enhancement is achieved. To verify the spatial evolution of the structural and chemical features before and after the deposition of Au NPs on graphene, we performed Raman mapping of the intensity of the G-band, as shown in Fig. 1 (g, h). Despite the deposition of Au NPs, no significant changes were observed, indicating that the Au NPs were nearly uniformly distributed on the graphene surface. Regarding potential applications, we evaluated the sensing capability of the as-prepared Au NPs/graphene system for detecting various analytes using SERS. Various analytes, such as R6G, MeB, Sudan I, and mixed dyes (R6G + MeB + Sudan I), were chosen, as shown in Fig. 2 (a, b). Typically, these analytes have numerous ring groups in their molecular structures that would interact with π bonding from the graphene. Consequently, Raman signals from the substrate were expected to primarily originate from the molecules of the target substance bound to graphene between the Au NPs, where the electric field enhancement is strongest. To validate this hypothesis, various SERS platforms, including SiO2, graphene/SiO2, and Au NPs/SiO2 were prepared. All analytes were diluted with DI water to prepare a 1×10-7 M solution. Target analytes at a concentration of 1×10-7 M were placed on each substrate by drying droplets, which were then dried to anchor the molecules on the substrate surface, as illustrated in Fig. 2 (c). To ensure comparability, all measurements were performed under the same conditions. The Raman spectrum results of each SERS platform are illustrated in Fig. 2 (d). Generally, R6G shows characteristic peaks at approximately 1127 cm−1 (C–H in-plane bending) and 1360, 1507 cm−1 (aromatic C–C stretching). MeB shows characteristic peaks at 1402 cm−1 (symmetric C–N stretches) and 1620 cm−1 (asymmetric stretching vibration of benzene rings). Sudan I dyes demonstrate characteristic peaks at approximately 1227 cm−1 (C–O stretching vibration and CCH scissoring bending of naphthalene ring), 1495 cm−1 (C=N, N–N stretching vibration, and N–H in-plane bending vibration), and 1596 cm−1 (C–C scissoring bending from the benzene ring and N=N stretching vibration). Comparing graphene/SiO2 with SiO2, we found that the Raman intensity of the analytes was enhanced by introducing graphene. This phenomenon can be attributed to the chemical interaction effect. Previous studies have reported that monolayer graphene facilitates charge transfer between graphene and probe molecules, resulting in a vibration-mode-dependent enhancement. Further, aromatic molecules tend to stack parallel to the π bonds of graphene through π–π stacking, leading to enhanced resonant energy transfer. By contrast, the Au NPs/graphene/SiO2 substrate exhibited significantly higher SERS sensitivity compared to the other control samples, as shown in Fig. 2 (e).
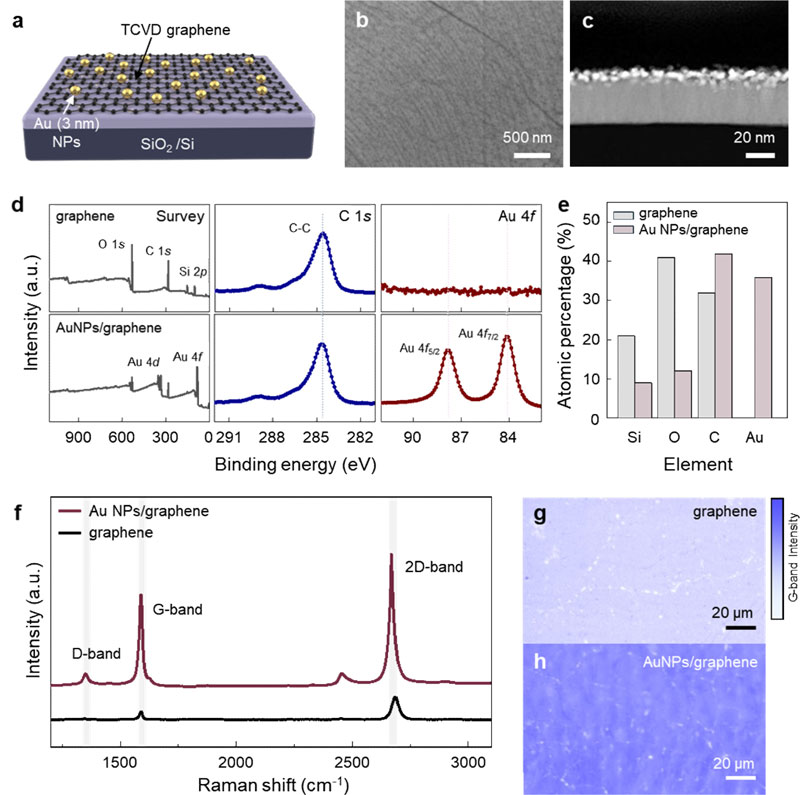
(a) Schematic illustration of the cross-section of Au NPs/graphene substrate. (b) Top-view and (c) cross-section SEM images of Au NPs/graphene substrate. (d) XPS survey, C 1s and Au 4f core-level spectra, and (e) the elemental atomic percentages collected from pristine graphene and Au NPs/graphene thin films. (f) Raman overview spectra and (g, h) spatially resolved Raman mapping of the G-band for graphene with and without Au NPs.
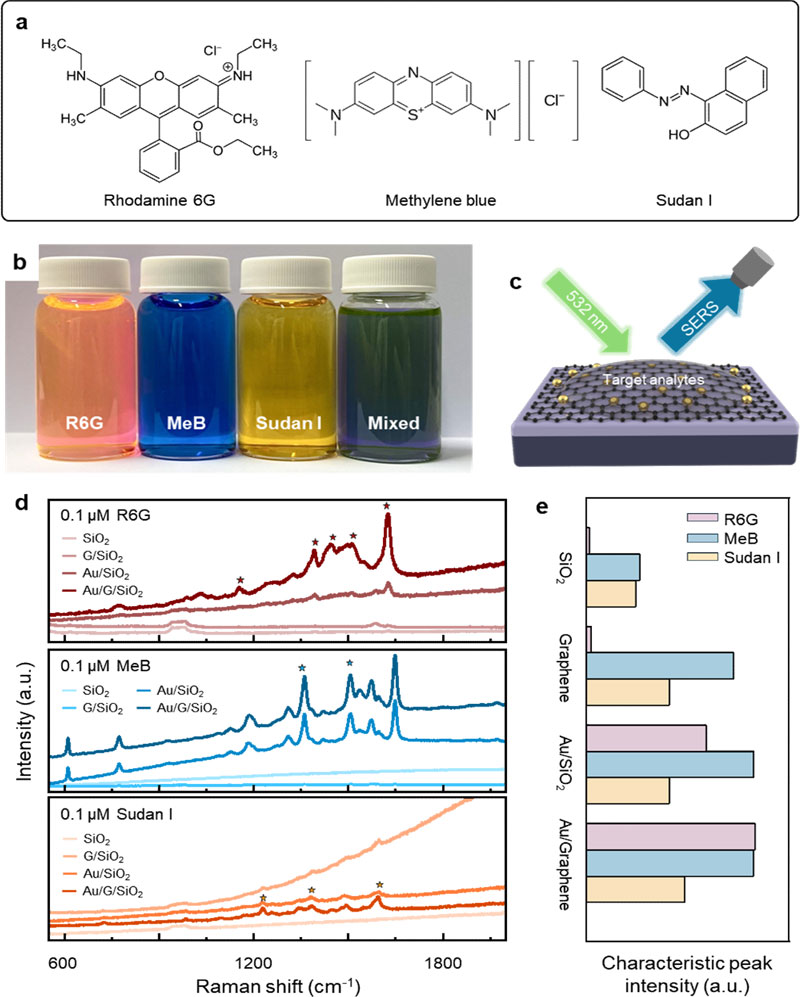
(a) Molecular structure illustrations of the dye molecules (R6G, MeB, and Sudan I). (b) Photograph of R6G, MeB, Sudan I, and mixed target analytes dispersed at a concentration of 1×10-7 M. (c) Schematic illustration of SERS measurements of analyte molecules on the SERS substrate. (d) Comparisons of SERS spectra and (e) main characteristic peak intensities for 1×10-7 M of R6G (red), MeB (blue), and Sudan I (orange) obtained from various SERS substrates.
By decorating Au NPs on graphene, multiple Au NPs are closely positioned, resulting in strong electric fields on the SERS platforms. The electromagnetic-coupled effect due to the aggregated NPs and the strong electrical interactions between Au NPs and graphene are responsible for the significantly enhanced Raman signal of the analytes. This strong enhancement of the localized electromagnetic effect from the Au NPs, coupled with additional chemical interactions from graphene, offers a highly advantageous opportunity for the characterization of adsorbed target molecules. These results can be attributed to the electromagnetic and chemical correlations between the Au NPs and graphene. Our approach, involving surface-engineered SERS platforms via complementary hybridization with Au NPs and graphene, demonstrates the potential for highly sensitive detection of π-conjugated molecules due to collaborative electromagnetic and chemical correlations.
Fig. 3 (a) illustrates the feature extraction process for applying various types of classification ML algorithms to the measured Raman spectra. First, to capture the distinct characteristics of the measured Raman fingerprint modes, customized data preprocessing steps were developed. These steps include denoising the raw Raman spectra, baseline subtraction, and normalization. The denoising process was performed using the Savitzky–Golay filtering algorithm, implemented using the SciPy library in Python. To capture the precise Raman characteristics, we performed baseline subtraction using polynomial baseline extraction from the Python pybaseline library. Sequentially, minimum-maximum normalization was implemented, scanning the data from 0 to 1. After pre-processing the original Raman spectra, we selected five representative Raman modes for each target analyte to apply Lorentzian curve fitting. Lorentzian curve fitting was performed using the curve-fit modules from the SciPy library. By appropriately optimizing the fitting parameters, we achieved Lorentz-fitted curves with r-squared values over 99%. This resulted in precise peak information, such as peak intensity, peak position, and peak width, which was used as ML feature data. By extracting the feature dataset for 100 Raman spectra (25 extracted feature sets each for R6G, MeB, Sudan I, and mixed dyes, totaling 100 sets), we prepared and reorganized the database for applying ML, with the feature datasets corresponding to the label data as the answer dataset. To validate the scheme for classifying the four different chemical analytes, we used a supervised ML approach. The classification was performed using well-defined training algorithms from Scikit-Learn in Python, such as linear discriminant analysis, decision tree, support vector machine (SVM), ensemble bagged trees, k-nearest neighbor (kNN), and neural network with k-fold (k = 5) validation, as illustrated in Fig. 3 (a). The classification results are presented as a confusion matrix, as illustrated in Fig. 3 (b). The prediction accuracies of the linear discriminant analysis, decision tree, SVM, ensemble bagged trees, kNN, and neural network were 88%, 95%, 83%, 95%, 93%, and 99%, respectively, as shown in Fig. 3 (c). The decision tree and ensemble bagged tree models exhibited slightly similar classification behaviors. Among them, the kNN model, which had the highest prediction accuracy, was used for further ML analysis. In addition, the incorrect labels were mostly distributed among the mixed analytes labeled as R6G and MeB, indicating that while the classification models are excellent, room for further optimization remains.
4. CONCLUSIONS
This study introduces a novel approach that harnesses the synergistic effects of graphene and Au NPs to significantly enhance Raman signals, thereby enabling highly sensitive SERS platforms for identifying diverse analytes. By capitalizing on collaborating electromagnetic and chemical interactions, our surface-engineered hybrid material offers an unprecedented level of sensitivity, paving the way for nondestructive and highly sensitive detection capabilities in SERS-based applications. In addition, we demonstrate an innovative strategy that leverages ML techniques to classify various analytes based on an extracted feature database. Through our approach, involving data preprocessing, extraction of essential feature data, and sophisticated evaluations employing k-fold (k = 5) validation, we demonstrated the high prediction accuracy of various ML models.
Our results demonstrated significantly higher sensitivity than conventional methods, indicating promising potential and applicability for molecular detection in the areas of medicine, food safety, and biotechnology. Additionally, the application of SERS analysis combined with ML algorithms assists in the quick and direct analysis and processing of data, making it suitable for molecular-level detection, which is challenging using traditional SERS technology. Furthermore, the high predictive accuracy of the ML models is expected to broaden the scope and depth of SERS applications in various fields.
Acknowledgments
J. H. C., and G. B. contributed equally to this study. This work was supported by the Technology Innovation Program (or Industrial Strategic Technology Program) funded by the Ministry of Trade, Industry, and Energy (20013138).
REFERENCES
-
M. Moskovits, “Suface-enhanced Raman spectroscopy: a brief retrospective”, J. Raman. Spectrosc., Vol. 36, No. 6-7, pp. 485-496, 2005.
[https://doi.org/10.1002/jrs.1362]
-
R. Petry, M. Schmitt, and J. Popp, “Raman Spectroscopy—A Prospective Tool in the Life Sciences”, ChemPhysChem, Vol. 4, No. 1, pp. 14-30, 2003.
[https://doi.org/10.1002/cphc.200390004]
-
B. N. Khlebtsov, D. N. Bratashov, N. A. Byzova, B. B. Dzantiev, and N. G. Khlebtsov, “SERS-based lateral flow immunoassay of troponin I by using gap-enhanced Raman tags”, Nano Research, Vol. 12, No. 2, pp. 413-420, 2019.
[https://doi.org/10.1007/s12274-018-2232-4]
-
J. Fei, L. Wu, Y. Zhang, S. Zong, Z. Wang, and Y. Cui, “Pharmacokinetics-on-a-Chip Using Label-Free SERS Technique for Programmable Dual-Drug Analysis”, ACS Sens., Vol. 2, No. 6, pp. 773-780, 2017.
[https://doi.org/10.1021/acssensors.7b00122]
-
S. Xu, J. Zhan, B. Man, S. Jiang, W. Yue, S. Gao, C. Guo, H. Liu, Z. Li, J. Wang, and Y. Zhou, “Real-time reliable determination of binding kinetics of DNA hybridization using a multi-channel graphene biosensor”, Nat. Commun., Vol. 8, No. 1, p. 14902, 2017.
[https://doi.org/10.1038/ncomms14902]
-
Q. Zhou, M. Jin, W. Wu, L. Fu, C. Yin, and H. Karimi-Maleh, “Graphene-Based Surface-Enhanced Raman Scattering (SERS) Sensing: Bibliometrics Based Analysis and Review”, Chemosensors, Vol. 10, No. 8, p. 317, 2022.
[https://doi.org/10.3390/chemosensors10080317]
-
B. N. J. Persson, K. Zhao, and Z. Zhang, “Chemical Contribution to Surface-Enhanced Raman Scattering”, Phys. Rev. Lett., Vol. 96, No. 20, p. 207401, 2006.
[https://doi.org/10.1103/PhysRevLett.96.207401]
-
A. Otto, “The ‘chemical’ (electronic) contribution to surface-enhanced Raman scattering”, J. Raman. Spectrosc., Vol. 36, No. 6-7, pp. 497-509, 2005.
[https://doi.org/10.1002/jrs.1355]
-
B. Sharma, R. R. Frontiera, A.-I. Henry, E. Ringe, and R. P. Van Duyne, “SERS: Materials, applications, and the future”, Mater. Today, Vol. 15, No. 1-2, pp. 16-25, 2012.
[https://doi.org/10.1016/S1369-7021(12)70017-2]
-
C. Guo, Y. Luo, R. Zhou, and G. Wei, “Triphenylalanine peptides self-assemble into nanospheres and nanorods that are different from the nanovesicles and nanotubes formed by diphenylalanine peptides”, Nanoscale, Vol. 6, No. 5, pp. 2895-2901, 2014.
[https://doi.org/10.1039/c3nr02505e]
-
X. Kong, Q. Chen, and Z. Sun, “Enhanced SERS of the complex substrate using Au supported on graphene with pyridine and R6G as the probe molecules”, Chem. Phys. Lett., Vol. 564, pp. 54-59, 2013.
[https://doi.org/10.1016/j.cplett.2013.02.006]
-
X.-M. Lin, Y. Cui, Y.-H. Xu, B. Ren, and Z.-Q. Tian, “Surface-enhanced Raman spectroscopy: substrate-related issues”, Anal. Bioanal. Chem., Vol. 394, No. 7, pp. 1729-1745, 2009.
[https://doi.org/10.1007/s00216-009-2761-5]
-
B. N. Khlebtsov, D. N. Bratashov, N. A. Byzova, B. B. Dzantiev, and N. G. Khlebtsov, “SERS-based lateral flow immunoassay of troponin I by using gap-enhanced Raman tags”, Nano Research, Vol. 12, No. 2, pp. 413-420, 2019.
[https://doi.org/10.1007/s12274-018-2232-4]
-
X. Ling, L. Xie, Y. Fang, H. Xu, H. Zhang, J. Kong, M. S. Dresselhaus, J. Zhang, and Z. Liu, “Can Graphene be used as a Substrate for Raman Enhancement?”, Nano Lett., Vol. 10, No. 2, pp. 553-561, 2010.
[https://doi.org/10.1021/nl903414x]
-
J. Lee, S. Shim, B. Kim, and H. S. Shin, “Surface-Enhanced Raman Scattering of Single- and Few-Layer Graphene by the Deposition of Gold Nanoparticles”, Chem. Eur. J., Vol. 17, No. 8, pp. 2381-2387, 2011.
[https://doi.org/10.1002/chem.201002027]
-
W. Xu, N. Mao, and J. Zhang, “Graphene: A Platform for Surface-Enhanced Raman Spectroscopy”, Small, Vol. 9, No. 8, pp. 1206-1224, 2013.
[https://doi.org/10.1002/smll.201203097]
-
L. Xie, X. Ling, Y. Fang, J. Zhang, and Z. Liu, “Graphene as a Substrate To Suppress Fluorescence in Resonance Raman Spectroscopy”, J. Am. Chem. Soc., Vol. 131, No. 29, pp. 9890-9891, 2009.
[https://doi.org/10.1021/ja9037593]
-
Z.-Q. Tian, B. Ren, and D.-Y. Wu, “Surface-Enhanced Raman Scattering: From Noble to Transition Metals and from Rough Surfaces to Ordered Nanostructures”, J. Phys. Chem. B, Vol. 106, No. 37, pp. 9463-9483, 2002.
[https://doi.org/10.1021/jp0257449]
-
X. Wang, W. Shi, G. She, and L. Mu, “Surface-Enhanced Raman Scattering (SERS) on transition metal and semiconductor nanostructures”, Phys. Chem. Chem. Phys., Vol. 14, No. 17, p. 5891, 2012.
[https://doi.org/10.1039/c2cp40080d]
-
A. Otto, “Theory of First Layer and Single Molecule Surface Enhanced Raman Scattering (SERS)”, Phys. Stat. Sol. A, Vol. 188, No. 4, pp. 1455-1470, 2001.
[https://doi.org/10.1002/1521-396X(200112)188:4<1455::AID-PSSA1455>3.0.CO;2-4]
-
W. Ren, Y. Fang, and E. Wang, “A Binary Functional Substrate for Enrichment and Ultrasensitive SERS Spectroscopic Detection of Folic Acid Using Graphene Oxide/Ag Nanoparticle Hybrids”, ACS Nano, Vol. 5, No. 8, pp. 6425-6433, 2011.
[https://doi.org/10.1021/nn201606r]
-
W. Xu, J. Xiao, Y. Chen, Y. Chen, X. Ling, and J. Zhang, “Graphene-Veiled Gold Substrate for Surface-Enhanced Raman Spectroscopy”, Adv. Mater., Vol. 25, No. 6, pp. 928-933, 2013.
[https://doi.org/10.1002/adma.201204355]
-
Y. Li. H. Yan, D. B. Farmer, X. Meng, W. Zhu, R. M. Osgood, T. F. Heinz, and P. Avouris, “Graphene Plasmon Enhanced Vibrational Sensing of Surface-Adsorbed Layers”, Nano Lett., Vol. 14, No. 3, pp. 1573-1577, 2014.
[https://doi.org/10.1021/nl404824w]
-
Y. X. Leong, Y. H. Lee, C. S. L. Koh, G. C. Phan-Quang, X. Han, I. Y. Phang, and X. Y. Ling, “Surface-Enhanced Raman Scattering (SERS) Taster: A Machine-Learning-Driven Multireceptor Platform for Multiplex Profiling of Wine Flavors”, Nano Lett., Vol. 21, No. 6, pp. 2642-2649, 2021.
[https://doi.org/10.1021/acs.nanolett.1c00416]
-
Y. Ding, Y. Sun, C. Liu, Q. Jiang, F. Chen, and Y. Cao, “SERS-Based Biosensors Combined with Machine Learning for Medical Application”, ChemistryOpen, Vol. 12, No. 1, 2023.
[https://doi.org/10.1002/open.202200192]
-
H. Zhou, L. Xu, Z. Ren, J. Zhu, and C. Lee, “Machine learning-augmented surface-enhanced spectroscopy toward next-generation molecular diagnostics”, Nanoscale Adv., Vol. 5, No. 3, pp. 538-570, 2023.
[https://doi.org/10.1039/D2NA00608A]
-
R. Beeram, K. R. Vepa, and V. R. Soma, “Recent Trends in SERS-Based Plasmonic Sensors for Disease Diagnostics, Biomolecules Detection, and Machine Learning Techniques”, Biosensors, Vol. 13, No. 3, p. 328, 2023.
[https://doi.org/10.3390/bios13030328]